Enjoying your data lake from your lakehouse
When it comes to data, businesses have trust issues.
According to a survey by TDWI, 64% of CIOs(1) believe data quality and management are the biggest barriers to unleashing the power of all the information they process and store.
To gain more control, companies have invested in cloud data warehouses and data lakes. The growing volume, variety, and array of data sources enterprises have to manage are testing the capabilities of both.
Some businesses have responded by turning to cloud data lakehouses, which merge elements of warehouses and lakes under one platform. The lakehouse model promises the best of both worlds, by blending technologies for analytics and decision making with those for data science and exploration.
As the name suggests, lakehouses are designed to provide a view across the entire data estate, to see the lineage and relationships attached to data and all the applications using it and have clarity over publish-subscribe data flows.
It’s a big step forward but still faces challenges before it can fully deliver. The lakehouse model needs data integration, data quality, and metadata management at an industrial scale.
Without the capability to govern data by managing discovery, cleansing, integration, protection, and reporting across all environments, lakehouse initiatives may be destined to fail.
Automating the data pipeline with AI
The stubborn resilience of manual processes is one of the most significant barriers to successful lakehouse implementation. Relying on techniques like hand-coding to build a data pipeline, for example, can limit scalability and create unnecessary bottlenecks. Manual ingestion and transformation of data is also a complex multi-step process that creates inconsistent, nonrepeatable results.
To deliver agility, speed, and repeatability, a lakehouse needs the data pipeline to be automated. Automation also suits the fast iteration and flexibility requirements of agile development, allowing changes to be made quickly and reducing the risk of bugs.
Automation becomes even more vital when data quality is on the line. Problems that aren’t caught early during ingestion can cause broader downstream issues. Business insights based on inaccuracies or inconsistencies between different data assets can result in flawed decision making.
With data volumes(2) surging, it is nearly impossible to manually spot all the potential data quality issues that can arise. In contrast, using AI to automatically detect signals of incomplete and inconsistent data using automated business rules can have a dramatic impact on the reliability of analytics.
Four cornerstones of cloud lakehouse data management
TDWI’s CIO survey also showed a clear majority (86%) believed that a systematic approach to data management is vital to the success of any data strategy.
Without it, enterprises will not be able to accelerate time to value, reduce costs, improve efficiency, increase scale, add flexibility, and deliver trusted insights for business decision making.
Those challenges aren’t new. But if not addressed, the same difficulties and mistakes that have characterized cloud data warehouses and data lakes will hobble cloud data lakehouse initiatives too.
Informatica and Capgemini recommend a four-step approach to help businesses avoid the data management pitfalls of the past.
01 Metadata Management. First, you need metadata management to effectively discover, classify, and understand how data is proliferating through your organization. Informatica Enterprise Data Catalog (EDC) can help you discover and inventory data assets across your organization. That includes business glossary and lineage data, so you know where data came from and what parts of the business connect to it.
02 Ingestion, curation, transformation, and sharing. Next, you need data integration. Data integration is more than simple intake; a best-of-breed solution supports all data ingestion and integration patterns. Mass ingestion of files, IoT streaming data, and database initial and incremental loads are vital requirements to hydrate your data lakehouse. Look for ETL/ELT and pushdown optimization to process data once it's in the cloud, ideally performed in a serverless elastic scaling runtime. You also need the broadest connectivity across clouds, SaaS, and on-premises applications.
03 Data quality. Embedding data quality enables you to deliver trusted data through comprehensive profiling, data quality rule generation, dictionaries, and more. Informatica Cloud Data Quality (CDQ) helps you quickly identify, fix, and monitor data quality problems in your cloud and on-premises business applications.
04 Data privacy and security. Lastly, data needs to be protected. When operating in co-located cloud environments, data access and use must be trusted. Applying data-centric protections such as data masking can help limit exposure to appropriate applications and users. This is even more critical in public cloud-hosted application environments, where multiple tenants can coexist on shared resources to increase risks.
Is it safe to migrate a lakehouse to the cloud?
Cloud economies enable organizations to manage workloads faster, taking advantage of elasticity but without the large opex investments required when rolling out digital transformation projects with in-house or on-premises resources.
But while cloud remains the future, too many organizations are held in the past by data protections that are hard-wired to legacy on-premises applications and systems. Every business considering taking the data lakehouse model to the cloud has to ask whether their new hosted environments can be trusted with business-critical information.
As a data owner and processor, businesses are responsible for ensuring safe data access and appropriate use in hosted applications. Cloud providers, however, don't typically extend privacy protections to the application and data layers.
A shared-responsibility model for cloud security can help alleviate these concerns.
CONCLUSION
To provide high-quality, actionable data to the business quickly, you need an automated lakehouse cloud data management solution that enables you to have a complete view of where all your critical data resides across different silos, applications, and regions.
With AI-powered and cloud-native data management from Informatica and Capgemini, you can safely leverage the lakehouse model to unleash the power of data warehouses and data lakes - for data residing inside and outside the enterprise.
1 TDWI Best Practices Report: Cloud Data Management
2 World Economic Forum: How much data is generated each day?
About Capgemini
A global leader in consulting, technology services and digital transformation, Capgemini is at the forefront of innovation to address the entire breadth of clients’ opportunities in the evolving world of cloud, digital and platforms. Building on its strong 50-year heritage and deep industry-specific expertise, Capgemini enables organizations to realize their business ambitions through an array of services from strategy to operations. Capgemini is driven by the conviction that the business value of technology comes from and through people. It is a multicultural company of almost 220,000 team members in more than 40 countries. The Group reported 2019 global revenues of EUR 14.1 billion.
Learn more about us at https://www.capgemini.com/
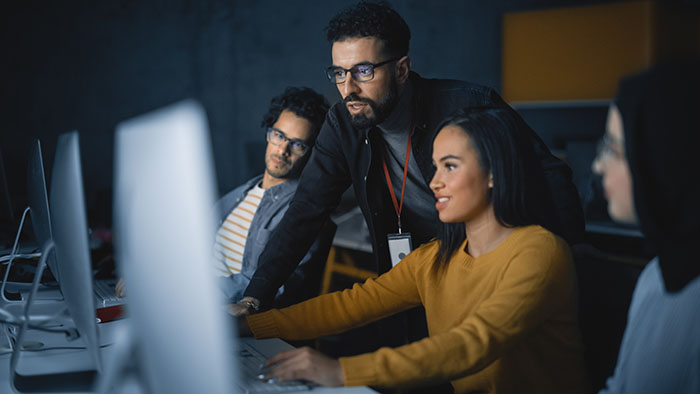
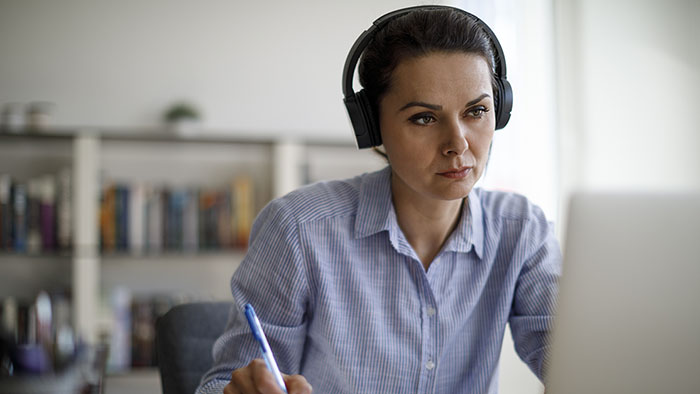
May 31, 2023
May 31, 2023
