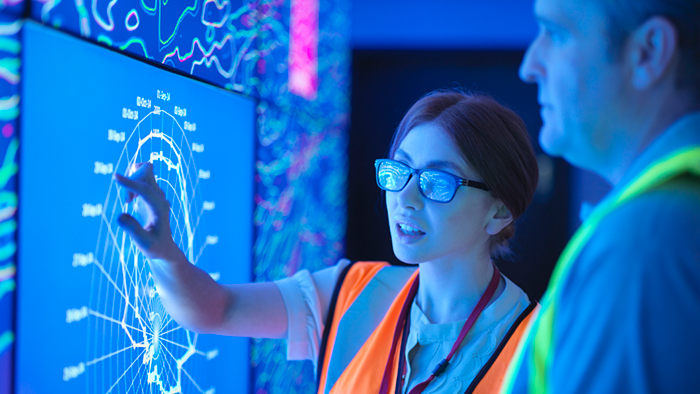
Data management is the implementation of policies and procedures that put organizations in control of their business-critical data, regardless of where that data resides. One of the most strategic assets for any enterprise regardless of industry is data, and data management is about creating trusted data to fuel innovation and decision-making.
Having to cope with duplicate and conflicting data from numerous sources across the enterprise, as well as from third-party sources, is a widespread problem. The associated costs can be very high. Also, because data changes over time, it’s easy for it to get out of sync and become fragmented, incomplete, inaccurate, and inconsistent. As data degrades, the people who use it lose trust.
Consider the impact on a sales call if an account manager accesses incomplete or inaccurate customer information. The wrong answer to any of these questions could put a new sale—or existing relationship—at risk:
- Is the location correct, or has the customer’s address changed?
- How confident is the account manager in knowing which products the customer owns and uses?
- Are there any open service items?
Data management principles and procedures can help by making sure data conforms to organizational best practices for access, storage, backup, retirement, and the controlled progression of data to and from each stage within its lifecycle.
Data management practices are also useful in rectifying conflict among data from new and duplicative data sources. Data management is the best way for organizations to provide access to accurate data when and where it is needed, without ambiguity or conflict, thereby avoiding miscommunication.
Well-managed data is also easy to access and searchable – across the enterprise. Data management includes modernizing data warehouses, data lakes, applications, and analytics in the cloud. The more complete and comprehensive your data management, the faster and more successful your cloud modernization initiatives will be.
It also minimizes the risk of noncompliance with data protection regulations and mitigates legal complications related to data.
Three important aspects of data management
Over the past decade, organizations have been shifting toward digital modernization. They have taken advantage of the cloud’s flexibility, agility, scale, and reduced costs by moving their infrastructure to the cloud to modernize applications, analytics, databases, data warehouses, and data lakes.
Organizations across industries need to run, interoperate, and support all combinations of multicloud and multi-hybrid environments. Second, they need to share, deliver, and democratize data across lines of business and across other enterprises. While doing so, organizations must also automate discovery and classification of sensitive data across sources, map sensitive data to business processes and regulations, and monitor data use and proliferation to comply with a rapidly expanding number of data privacy regulations globally.
While organizations are heading down the path of delivering a complete view of data, many of them still lack a unified, 360-degree view of their business-critical data across business units, departments, and systems. They need comprehensive, intelligent solutions that automate thousands of manual tasks and accelerate data-led transformations by applying artificial intelligence (AI) and machine learning (ML) to data and metadata to ensure consistent data quality and end-to-end data governance across the enterprise.
Some of the most significant challenges holding organizations back from accelerating true digital transformation include the exponential growth of data, fragmentation of data and decentralization across multiple clouds and hybrid environments.
Organizations require data management that:
- Integrates data from all sources: Handle the complex challenges of dispersed and fragmented data on any platform and any cloud, including multicloud and multi-hybrid environments.
- Delivers a 360-degree view of enterprise data: Gain a single, trusted view of all business-critical data across the enterprise to create new and differentiated experiences for customers, suppliers, employees, and partners.
- Implements data governance to support self-service analytics: Ensure the trustworthiness of data with data governance and data privacy as strategic requirements to power real-time insights, reduce security breaches and ensure compliance with data privacy regulations.
What processes are involved with data management?
To manage data successfully, organizations must have processes and controls in place to ensure that as data sources are added, the data is transformed into a reliable and trustworthy enterprise asset. The processes must also enable the seamless integration of dispersed and fragmented data on any platform and any cloud, including multicloud and multi-hybrid environments.
A common approach to data management is to use a master data file called master data management (MDM). MDM creates a master record (also known as a “golden record” or “best version of the truth”) that contains the essential information about master data domains—a customer, location, product, supplier, and so on—to facilitate a task or action such as a marketing campaign, service call, or sales conversation.
Data managed as an enterprise asset also requires data governance and the imposition of data quality rules. Data governance eliminates ambiguity regarding what data means and
ensures the nuances are documented and understood, and that data is shared with authorized users. Data quality ensures the data itself is understood, complete, formatted correctly, and suitable for the intended use (often called “fit for purpose”).
Equally important to managing data successfully is enterprise-scale automation with AI to identify, match, and merge data across the systems that hold it, so that clean, reliable, trustworthy data is shared with the modern applications, systems, and cloud-based analytics that need it. Organizations simply do not have enough time or people to manage the overwhelming amount of data without AI-driven automation.
These are key steps within data management:
- Discover: Locate untapped sources of relevant data, even when they aren’t obvious or easy to find.
- Acquire: Connect to many disparate data sources and heterogeneous applications from across departments, lines of business, brands, and elsewhere at varying latencies based on business requirements.
- Cleanse and enrich: Execute data quality and data enrichment capabilities to improve the overall quality of data.
- Relate: Amplify the value of data by capturing where the data came from, understanding its relationship to other data, and creating an audit trail of changes to data.
- Reason: Infer and identify insights hidden in any type of data with delivery based on the context of the user.
- Secure: Ensure data conforms to business rules, which helps enforce regulatory compliance, data protection and privacy requirements.
- Deliver: Provide data to the right users, supporting the right processes at the right time in the right context.
What are the benefits of data management?
With data management, organizations can create one source of truth for enterprise data, eliminating redundancies and confusion that can occur with multiple, conflicting sources of data. For example, data management ensures that when customer contact information changes, the organization will not attempt sales or marketing outreach using both the old and new information.
The following are some business-critical benefits of data management:
- Enhance workforce productivity through self-service data access
- Boost revenue and profitability with more accurate AI models
- Increase agility with 360-degree views of data across the enterprise
- Drive operational efficiency with simplified workflows
- Increase access to data on any platform, any cloud, and for any type of user in multicloud and multi-hybrid environments
- Protect data privacy, reduce risk exposure and ensure regulatory compliance
- Improve customer experience by connecting trustworthy data to create personalized engagement, increase cross-sell and activate next best action
- Optimize supplier relationships to ensure compliance and better assess performance and risk
- Create an omnichannel product experience across all channels
Data management solutions
Over the past few years, AI and ML have been applied to the following three categories of data management solutions making it possible to perform activities that in the past were simply too complex and manually intensive to be done at enterprise scale.
The three categories of data management solutions are:
Data warehouse, data lake and application modernization—Automates analytics in the cloud with AI-powered, cloud-native data management for all users and all data in multicloud and multi-hybrid environments.for all users and all data in multicloud and multi-hybrid environments.
- Integrates and provides real-time connectivity across cloud-native and enterprise applications to manage business processes
- Manages data complexity and delivers a connected experience across cloud and on-premises environments
Master data management (MDM)—Enables intelligent discovery and understanding of all data within and outside of the enterprise, accesses and ingests all types of data wherever and whenever required, curates and prepares data in a self-service fashion, so it is fit for purpose, and delivers a unified, authoritative, 360-degree view of all enterprise data.
- Builds stronger customer relationships by enabling complete, contextual, and trusted customer profiles
- Delivers trusted, relevant, and detailed product content across digital touchpoints
- Automates the management of supplier information to streamline business processes and workflows
Data governance and privacy—Governs and protects data across—and beyond—the enterprise, with automation to ensure that reliable, trusted data is at the core of every decision, and to decrease data risk exposure.
- Makes data available to all users—technical and non-technical alike—to empower employees to make data-driven decisions confidently
- Delivers safer data for use by operationalizing a complete, data privacy framework to lower data risk
- Applies regulatory compliance best practices to reduce risk and safeguard data trustworthiness across the enterprise
Learn about the Informatica Intelligent Data Management Cloud.
Data management in your industry
Every industry has unique data management challenges, based on business requirements specific to a particular market. However, regardless of their industry, all organizations need to innovate to stay competitive. The ability to scale resources in an agile manner, achieve trusted data insights and protect sensitive data are critical to success.
Let’s look more closely at the importance of data management within various industries.
Data management and healthcare
The healthcare industry has massive amounts of highly complex data residing across a breadth of diverse, multi-hybrid systems. AI-driven automation is the only way to get value from it and manage it effectively.
Healthcare is becoming more data-driven, collaborative, and patient-centric. These trends have led to a greater adoption of cloud-based services. To address the critical challenges in accelerating digital transformation, healthcare organizations also must harness AI and ML to gain real-time insights from data to drive innovation and manage the scale and complexity of healthcare data itself.
Healthcare organizations require modern data management and governance tools and technologies designed to ease the discovery, ingestion, preparation, analysis, and sharing of vast amounts of data. These tools empower healthcare organizations to:
- Enable the seamless exchange of compliant healthcare data
- Manage healthcare data as a reliable, trustworthy asset
- Discover, classify, and protect sensitive patient data
- Consolidate data for in-depth patient insights
Data management and the public sector
Public sector agencies need to achieve comprehensive visibility, situational awareness, and understanding of the data they currently possess—in addition to all the data still to come. To successfully manage public sector data, agencies need the right technology and processes.
Traditional manual processes simply cannot efficiently and effectively manage all the data at scale, while also ensuring data quality and governance. Instead, public sector agencies need to deploy automation in their data management strategies.
Data management in the public sector requires solutions that:
- Identify data created or collected from authoritative sources—wherever it resides
- Create a trusted, unified, real-time view of individual citizens, communities, and neighborhoods—so employees can address emergencies and deliver new citizen services
Policy makers, city managers, urban planners and other public sector professionals require tools to create a holistic view of all data, including its lineage and relationship views, to profile and assess it—and produce reliable data quality metrics. These solutions must also employ AI, data cataloging, and monitoring to automate protection of citizen data in compliance with fast-changing data privacy regulations.
Data management and manufacturing
To make transformative business decisions, manufacturers need quick, easy access to trustworthy, reliable data about products, customer demand and risks to their supply chains. In the case of supply chains, manufacturers frequently partner with multiple suppliers and sub-suppliers in different regions and must often deal with supplier data spread across multiple, siloed systems, and multi-hybrid applications spanning business units. This makes it difficult to achieve a single, integrated view of supply chain data. As a result, supply chain data is often inaccurate, inconsistent, incomplete, and fragmented, creating a huge data-management overhead and increasing costs for manufacturers. These data management challenges hinder a manufacturer's ability to digitally transform their supply chain operations.
Manufacturers require intelligent data management solutions at every level of the manufacturing process to achieve a single, unified view of supplier, product, and customer master data that will ensure global consistency and quality while respecting local and global compliance requirements.
Switching to a more accurate view of order effectiveness with intelligent data management would also:
- Ensure supply chain traceability, transparency, and risk assessment
- Reduce working capital
- Help evolve new business models
- Maintain a meaningful, high-touch relationship to encourage customer loyalty
Data management and financial services
Data management is critical to financial services companies to ensure every business unit, application, and employee has access to timely, trusted, transparent, and secure data to:
- Enhance the customer experience
- Expand wallet share
- Strengthen risk management and compliance
- Combat fraud
- Accelerate value from mergers and acquisitions
The industry has been accustomed to hiring more people to address data management challenges. This approach has led to inconsistent practices, processes, and controls, often resulting in higher losses from fraudulent transactions, increased credit risk exposures, lower responses to cross-sell marketing campaigns, and delays in onboarding new clients.
Modern, cloud-native data management tools are helping financial services companies achieve transformative breakthroughs in speed, resiliency, efficiency, and customer satisfaction—while ensuring data is trusted, accessible, and ready to be put to strategic use. “Data-ready” financial services companies implement intelligent data management to extract the highest business value from the highest-quality data—turning information into tangible results.
Customer success stories
FDA uses modern data architecture to bring safe, high-quality, healthcare products to market
The U.S. FDA Center for Drug Evaluation and Research (CDER) promotes and protects public health by ensuring the availability of high-quality, safe, and effective drugs throughout the entire drug lifecycle.
Using Informatica’s Master Data Management software (MDM)—a part of the Intelligent Data Management Cloud that provides a single, consolidated, and reliable view of mission-critical data, such as individuals and organizations, events, and other key domains, as well as the relationships between data—CDER gained a 360-degree view of the global drug supply chain. In short, the organization can now holistically track the entire drug lifecycle—from inception to product. This empowers the agency to trace any potential harm, save lives, and respond to changes in supply and demand more quickly.
UNC Health implements data governance to deliver coordinated response to COVID-19
UNC Health is a not-for-profit integrated health care system owned by the state of North Carolina comprising UNC Hospitals and its provider network, the clinical programs of the UNC School of Medicine, and 12 affiliate hospitals and hospital systems across the state.
UNC Health wanted to provide self-service analytics from its data warehouse for business users and develop a comprehensive data stewardship framework. The organization used Informatica Axon Data Governance to provide employees and partners with a collaborative, shared view of data assets. And, Informatica Enterprise Data Catalog helps employees understand data lineage and expedite impact analysis.
AXA XL uses data engineering to streamline customer product offering
AXA XL is a global insurance and reinsurance company serving clients in over 200 countries through more than 100 offices on six continents. Following M&A-fueled growth, AXA used Informatica Enterprise Data Catalog to make data actionable and easy to find. They also used Informatica Data Engineering Quality to integrate, govern, and cleanse data from on-premises and cloud sources.
Rockwool integrates data from cloud and on-premises sources to drive digital transformation
Rockwool International is a leading manufacturer of stone wool solutions covering building, industrial, technical insulation, and customized solutions for wall and facade systems. The global business set out to solve its data management challenges by integrating disparate systems across 45 manufacturing facilities and offices in 39 countries. Using Informatica Cloud Integration Services, Rockwool accelerated digital transformation by improving data flows and information consistency throughout the company.
Move from digital modernization to true digital transformation
Whether you are embarking on cloud modernization, reimagining the customer experience with a unified, 360-degree view of data across your business or implementing enterprise data governance and privacy, intelligent and automated data management is key to driving your organization’s digital transformation. Learn about the AI-powered Intelligent Data Management Cloud.