Why Chief Data Officers Should Manage Data as a Product
Chief data officers (CDOs) play an ever-more crucial role in their organizations. No longer just guardians of compliance, they now shepherd key data initiatives such as delivering faster time to value from data, providing self-service access to data, and ensuring data is governed and of high quality for analytics and AI. But economic uncertainty, spiraling global regulations and growing technology stack complexity are compelling CDOs to seek out new ways to combat these market pressures.
We recently met virtually with members of the Informatica CDO Executive Advisory Board (EAB) to discuss these and other trending topics, such as data products and generative AI. We were joined by special guest Sanjeev Mohan, Principal at SanjMo advisory services and former Gartner Research VP, Big Data and Advanced Analytics. Sanjeev and the EAB members discussed how managing data as a product can help CDOs address the head winds they’re facing. To help explain why that approach can help, Sanjeev first looked at the data landscape.
The Drive Towards Decentralization with Data Mesh
The business need for more agility and self-service is driving the adoption of decentralized data approaches such as data mesh. Data mesh involves four principles, according to Sanjeev:
- Domain ownership
- Data as a product
- Self-service data infrastructure
- Federated computational governance
There are many advantages to implementing data mesh. It uses a domain-driven design, increases accountability, and helps alleviate a data engineer skills shortage. It also helps improve agility and potentially reduce data silos and redundancy.
But a data mesh architecture isn’t for everyone. For one thing, it lacks “how-to” guidance. In addition, many domain owners may lack the will and/or the skill to own data infrastructure. It also may lead to new data silos. And, it can be difficult to govern shared data.
8 Key Attributes of Data Products
As mentioned above, data products are a key part of implementing data mesh. While data products are closely associated with data mesh, according to Sanjeev they provide value independent of the data management approach adopted by organizations. He describes a data product as the codification of rules, context and semantics on data and metadata.
A data contract codifies the data product’s specifications, such as API access, quality, discoverability, availability, reliability, SLAs and more. It bridges a single data producer with multiple disparate consumers, so they have a common understanding. It removes ambiguity and second guesses.
Data products, said Sanjeev, have eight key attributes:
- Valuable
- Secure
- Discoverable
- Addressable
- Understandable
- Trustworthy
- Natively accessible
- Interoperable
Several EAB members who are already on this journey towards adoption of data products shared some best practices. One EAB member stated that a data product can be whatever you want it to be. Any type of data asset can be a data product, such as a dashboard, report, customer table, etc. The key is to decide how you are going to approach, design and use data products, applying product management principles. In other words, define how you are going to get a product experience.
Another EAB member described having a hierarchical structure with data foundations for finance, marketing, corporate, etc., which creates a mesh architecture that is shareable with access controls. On top of that, they have data marts, he said, with every data mart being a data product that is consumable by all, but the foundation is accessible only to select teams.
There is also the concept of a data product manager (not to be confused with a data steward). One EAB member mentioned having an organizational structure similar to product development teams, with a data product leader managing multiple data product managers covering a portfolio of data products.
Shift in Thinking and Approach
Implementing a data mesh and data product approach calls for a shift in thinking, Sanjeev declared. Many organizations currently prioritize technology first, with a narrow, bottom-up focus. This data-driven mindset can lead to technical debt, requires heavy data engineering resources, and pushes data quality downstream, often resulting in duplication and data silos.
A modern architectural approach to data products, on the other hand, is driven by business outcomes and focuses on process and self-service. It relies on a unified metadata plane to address discoverability, common understanding, governance, privacy and sharing of data.
Sanjeev noted that organizations taking a data products approach can see a 30% reduction in total cost of data ownership, according to research by McKinsey & Co.1
Assessing the Use of Large Language Models in a Corporate Setting
For the final portion of the discussion, the group shifted their attention to large language models (LLMs) and the implications of generative AI for data products. According to Sanjeev, LLMs are currently excellent at language prediction, but not reasoning. Trained on vast amounts of public data, they enable companies to be “gen AI first.”
There are drawbacks to LLMs too. They focus on unstructured, rather than structured, data, and are trained in batch mode, which can be slow and expensive. Asking questions is tricky; hence the rise of prompt engineering.
Sanjeev also introduced the concept of private or enterprise LLMs, but EAB members voiced concerns about privacy, stating that they don’t put corporate data into LLMs. In response, I recommended a tiered approach, in which the best of public LLMs with broader domain metadata is combined with private, company-only metadata.
EAB members agreed that questions around data privacy and protection are valid top of mind concerns at their organizations.
Next Steps
If you’d like to further explore topics that are top of mind for CDOs, check out our CDO page at www.informatica.com/cdo.
1Mckinsey-tech-trends-outlook-2022-next-gen-software
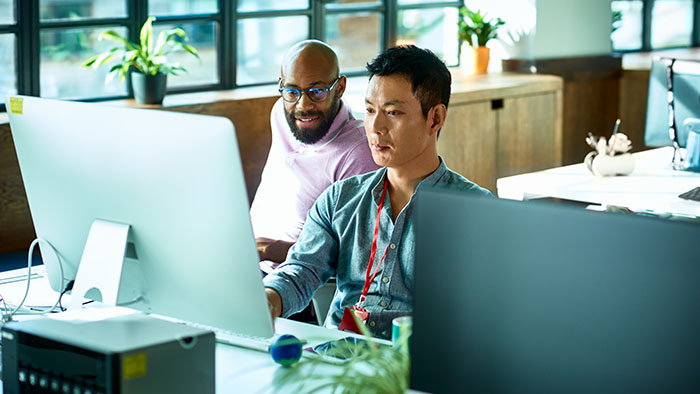
Aug 17, 2023
Aug 17, 2023

